|
|
|
The Chemist Volume 93 | Number 1 |

|
|
Selection of Influential Factors on Fume Hood Containment Performance Based on Questionnaire Survey and Fuzzy Comprehensive Evaluation
|
|
Abstract: There are multiple influential factors affecting fume hood containment performance in real laboratory environments and it is of great importance to prioritize these factors for further analysis of their impacts. Without a systematic approach, it is a common practice for researchers to select target influential factors based on their experience or even randomly, consequently imposing heavy financial burdens on themselves and increasing the environmental footprint. This interdisciplinary research aimed to propose a systematic and optimized approach for selecting influential factors of fume hood containment performance evaluation through questionnaire survey and fuzzy comprehensive evaluation. The questionnaire survey collected basic information of influential factors on fume hood containment performance, and then fuzzy comprehensive evaluation (FCE) was conducted in order to prioritize and select critical factors. The results of this study suggest that thermal challenge and inside clutter have significant fuzzy membership (84.5% and 89%, respectively) among all the influential factors and might be considered for further analysis of their impacts on fume hood containment performance. Proper selection of influential factors is vital to reducing the volume of the greenhouse gas emission during field test as well as the computational efforts in numerical simulation for hood performance evaluation. Further, the approach adopted in this study can also be referred to by industrial hygienists and ventilation engineers to determine the optimal methodology for fume hood containment performance evaluation.
Key Words: fume hood, influential factors, containment performance, questionnaire survey, fuzzy comprehensive evaluation, laboratory environment
*Corresponding author
|
|
|
Introduction
The fume hood has been adopted as a primary exposure control device given its ubiquitous presence in research laboratories (See Figure 1 for its profile and key features [1]).
It provides the widest range of protection from hazardous emission scenarios that include small to very large quantities of low to extremely hazardous materials generated at rates from less than 0.1 lpm to as much as 10 lpm [2].
 |
Figure 2 shows a diagram representing the increasing hazard level and generation potential (rate and quantity) on the axis Y and axis X, respectively [2]. Different types of exposure control devices (ECDs) shown on the diagram are utilized according to the matrix of hazard level and generation potential. Scientists and researchers working in laboratories mainly rely on robust fume hood performance to protect themselves from overexposure to hazardous airborne chemicals generated during experimental activities. This is critically important in case highly hazardous materials, e.g., ototoxicants or active pharmaceutical ingredients (APIs), are handled in the fume hood along with excessive noise exposure [3-5]. With the increasing complexity of laboratory experimental process, fume hood containment performance is affected by a wider variety of internal and external influential factors. According to ASHRAE standard 110 Methods of Testing Performance of Laboratory Fume Hoods, three alternate ratings can be determined depending on the purpose and condition of the test, namely “as manufactured” (AM) test, “as installed” (AI) test and “as used” (AU) test. While the AM test and AI test are aimed to evaluate the original hood design and installation integrity, respectively, the AU test investigates fume hood containment performance under the influence of real operational factors, which may include hood clutter, thermal challenge, contaminant emission character, maladjustment of the baffles, design and operation of the general ventilation systems, etc. [1]
Previous Research of Influential Factors on Fume Hood Containment Performance
The fume hood containment performance will be degraded by negative impacts from both internal and external influential factors, which leads to potential leakage of contaminants, and personal overexposure to hazardous materials or indoor air pollution, consequently [6-9].
In a recent research, Chen et al. explored the relationship between fume hood containment failure and three common influential factors through comprehensive numerical simulation with verification of field test [10]. The authors concluded that the tracer gas emission character seems to be a more dominant factor that contributes to hood containment failure, compared with the thermal challenge and inside clutters. However, this up-to-date research does not consider selecting multiple influential factors in a systematical way. In previous studies, Johnston et al. [11,12] linked thermal load generated by meeker burners and higher breathing zone concentrations of tracers in a positive linear relationship, while Ahn et al. [13] proposed a novel experimental method to explore the feasibility of using carbon dioxide (CO2) as an alternative tracer gas to analyze the relationship between sash opening heights, thermal loads, hood clutters and leakage values. A systematic review of quantitative experimental studies on factors affecting fume hood performance was conducted by Ahn et al. [6] and the review summarized that the distance between the emission point and breathing zone, the presence of a mannequin or real human subject, and the height or space of sash opening are the strongest potentials among all the influential factors on the performance of laboratory fume hoods. The importance of studying the effect of all these factors in a systematic and comprehensive manner was emphasized by the authors. He and Steven [14] investigated the interactions between the hood face velocity and the indoor cross-draft airflow velocity and underlined that these factors have significant effects (p <0.05) on hood containment performance, namely log-transformed breathing zone concentrations of the tracer gas. In their study, both the collar and bottom flange posed a negative impact on reducing manikin exposures, but such kind of impact can be mitigated through proper design of the hood sash.
Although the field investigation (e.g., face velocity measurement and flow visualization) seems to be practical and effective in hood performance evaluation, only several influential factors were considered in the research above because it will be cost-ineffective, time-consuming, or even impossible to evaluate all the potential factors in one single experimental study. On the other hand, computational fluid dynamics (CFD), as an emerging technology, has been widely adopted by research for quantitative evaluation of fume hood performance thanks to the increasingly improved computational capability. Michele and Alessio [15] proposed a CFD numerical procedure to analyze the effect of blockages in the sash area on hood containment performance. This novel research defined an equation to address viscous-induced effects, including boundary layer separation, lazy airflow and recirculation. This improved Dalla Valle Equation well describes the capturing velocity decay in front of the hood and shows a good fit with the results of numerical simulation, allowing a maximum deviation of 17.3 %. Another CFD study conducted by Hu et al. [16] highlighted that the location of the exhaust duct should not be located too close to the sash of the fume hood and the contaminant leakage is most likely to occur near either the bottom of the sash or the floor of the fume hood. Such conclusions are in line with the experimental findings by Fletcher and Johnson [17,18]. Other studies have also reported the promising use of CFD in assessing and quantifying the impact of influential factors on laboratory fume hood containment performance. Sangrok et al. [19] performed an initial research that calculate the leakage rate of the fume hood in order to determine a factor for workplace exposure assessment. The leakage rate derived from the numerical simulation is 3% with face velocities between 0.1 and 1.0 m/s at the sash opening, which provides fundamental data for evaluating the personal exposure dose during the processes of dispensing, dilution, and preparation of the radioisotopes. Through CFD simulation, Hu et al. [20] found that the level of contaminant leakage was related to not only the volume of the recirculating airflow behind the hood sash, but also the blockage caused by the baffles. Wherever the tracer ejector was placed, the level of the tracer leakage close to the bottom of the sash was the highest along the working aperture. These findings derived from numerical simulation are in consistent with previous experimental observations [18], which makes CFD modelling a good alternative to performing costly field experiments. While still incomplete, the growing body of evidence indicates that numerical simulation could be considered in laboratory fume hood performance evaluation at least as a supplementary method to physical measurements [21-26].
The research mentioned above have revealed the relationship between various influential factors and fume hood contain-ment performance, either qualitatively or quantitatively. Nevertheless, none of these studies adopt a systematic and optimized approach in selecting influential factors for hood containment performance evaluation. Currently, there are no pertinent meth-odologies and practices available in the literature. Therefore, the aim of this paper is to develop a new method for prioritizing and selecting critical influential factors on fume hood containment performance using ques-tionnaire survey and fuzzy comprehensive evaluation.
Design and Delivery of the Survey Instrument
Based on the literature review in the prior section, a combined (open-ended and close-ended) survey questionnaire was constructed and delivered to representative fume hood end-users in order to collect basic information of fume hoods used on site and potential improvement opportunities of safe application. An email-back questionnaire is utilized as the survey delivery mechanism in this research. This type of survey provides an opportunity to reach a much broader audience rapidly than many other survey methods, though the potential response rate might be lower. In addition, it is generally less expensive than a telephone survey, and participants can have a better understanding of the questions with adequate response time.
The questionnaire consists of four main sections: (1) background information regarding application of fume hoods; (2) multiple influential factors with three levels of weight for the participants to rank; (3) methodologies for fume hood containment performance evaluation; and (4) potential improvement opportunities for safe application of fume hoods. Detailed questions are exemplified in Table 1 and all of them are written in a neutral and non-leading way for respondents in order to prevent their responses from being biased toward any predefined ideas [27,28]. Thus, the variety of perspectives can be maximized. Before formal delivery of the survey, the questionnaire was pilot tested to three environmental health & safety professionals, two fume hood application experts, and one laboratory ventilation engineer who has been working with fume hoods for more than ten years. The participants in this beta test were asked to provide comments in terms of contents, delivery methods, the feasibility and comprehensibility of the survey, etc. [29]. This panel of multidisciplinary experts reported that the survey questions were easy to understand for both professionals and practitioners. They also suggested that delivering the survey through email would enable the participants to respond in a clear and prompt way. After minor revisions, the survey questionnaire was then distributed to 52 research institutes, universities and industrial companies, individually, and administrated within two months for collection of their responses.
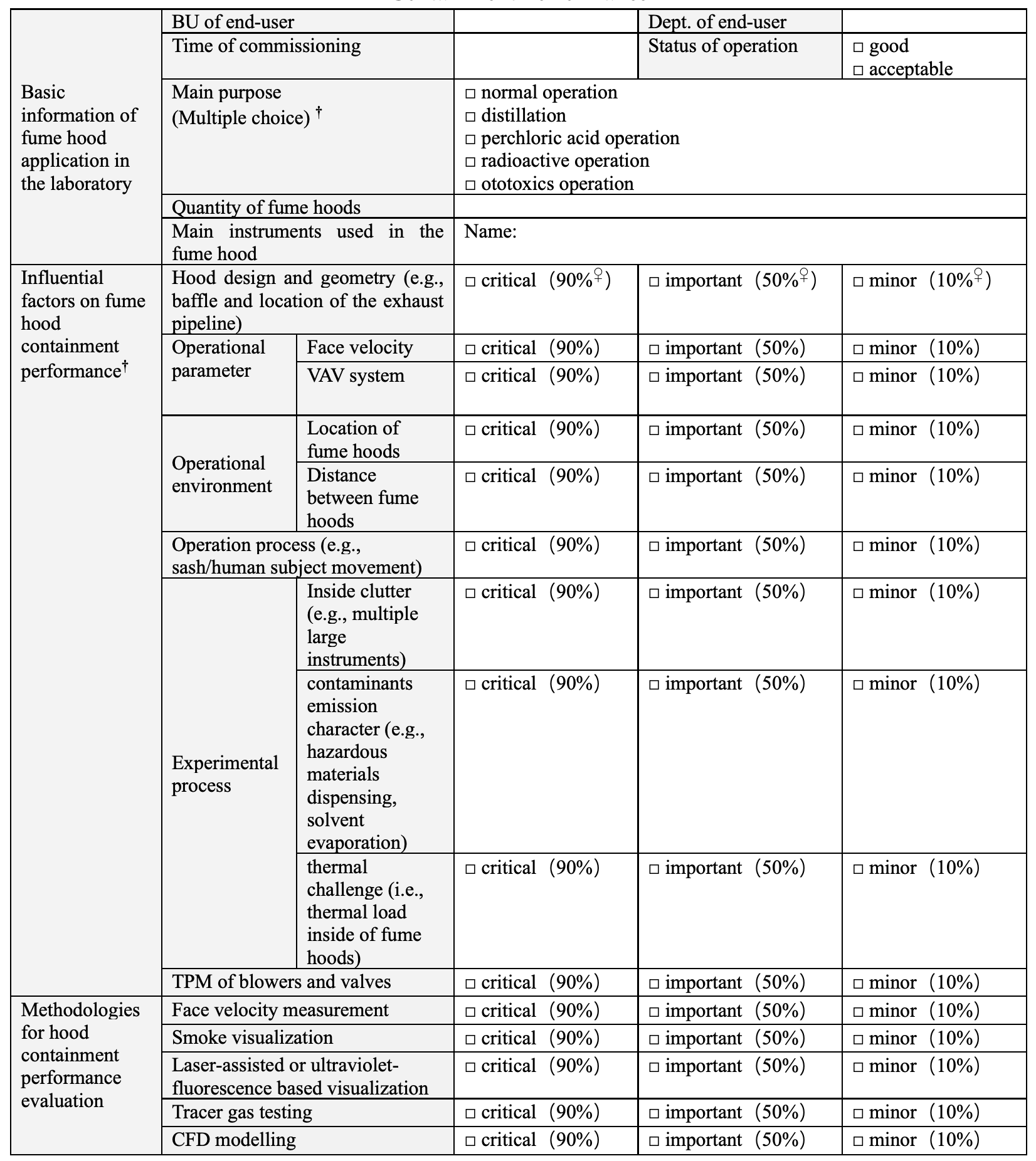 |
 |
With the joint efforts, 39 questionnaires were sent back finally from the survey participants and the feedback rate is relatively high considering the limited time resource. The information collected from the questionnaire survey supports selecting critical fume hood performance influential factors in the following sections, and might guide methodologies for hood containment performance evaluation in future studies as well.
Fuzzy Comprehensive Evaluation of the Influential Factors
In this section, there are two major steps in the selection of critical influential factors on fume hood containment performance, namely preliminary statistical analysis and fuzzy comprehensive evaluation. The first step is a screening process which is aimed at prioritizing relatively important items among a great variety of influential factors as specified in the survey questionnaire. The selectivity (S) of each influential factor was determined by the following equation (1):
where: q1, q2, q3, the number of questionnaires in three membership degrees, respectively; Sj, the selectivity of each influential factor in the questionnaire; w1, w2, w3, the percentage of weight (membership degree) in three categories (90%, 50% and 10%, respectively) for each influential factor in Table 1;and n1, n2, n3, the sum of influential factors selected by survey participants under three membership degrees for each influential factor.
For example, 29 participants considered hood design and geometry as a critical influential factor to be analyzed, 8 participants regarded it as an important factor, while 2 participants marked it as a minor factor only. Therefore, for this influential factor (hood design and geometry), its selectivity is calculated as: S1= 0.9×29 + 0.5×8 + 0.1×2= 30.3. Similarly, the selectivity of all the other influential factors in the questionnaire can be obtained as below:
S22= 0.9×25+0.5×14+0.1×0= 29.5;
S33= 0.9×25+0.5×8+0.1×6= 27.1;
S4= 0.9×20+0.5×15+0.1×4= 25.9;
S5= 0.9×22+0.5×9+0.1×8= 25.1;
S6= 0.9×30+0.5×7+0.1×2= 30.7;
S7= 0.9×32+0.5×5+0.1×2= 31.5;
S8= 0.9×36+0.5×3+0.1×0= 33.9;
S9= 0.9×32+0.5×6+0.1×1= 31.9;
S10= 0.9×29+0.5×10+0.1×0= 31.1
Followed by the calculation of the selectivity above, five relatively important influential factors have been screened out as contaminants emission character (S8), thermal challenge (S9), inside clutter (S7), TPM of blowers and valves (S10) and operation process (S6).
Secondly, fuzzy comprehensive evaluation (FCE) of the influential factors screened out was implemented thereafter in order to improve cost-effectiveness of fume hood containment performance analysis. The FCE method was proposed initially by Zadeh in the 1960s [30-31] and has been widely applied in hierarchical cluster analysis (HCA) for interdisciplinary studies including but not limited to biological genetics, information science and control engineering [32-34]. It is extremely useful in evaluation of interactive factors or factors that cannot be quantified directly [35]. Through fuzzy transformation and comprehensive evaluation, the integrated selectivity of each factor was obtained and the whole process became less subjective as the evaluation system is more complicated and comprehensive. Finally, two influential factors were identified further as critical research objects in fume hood containment performance evaluation. Detailed evaluation and calculation process is illustrated in Figure 3 and explained in the following paragraphs.
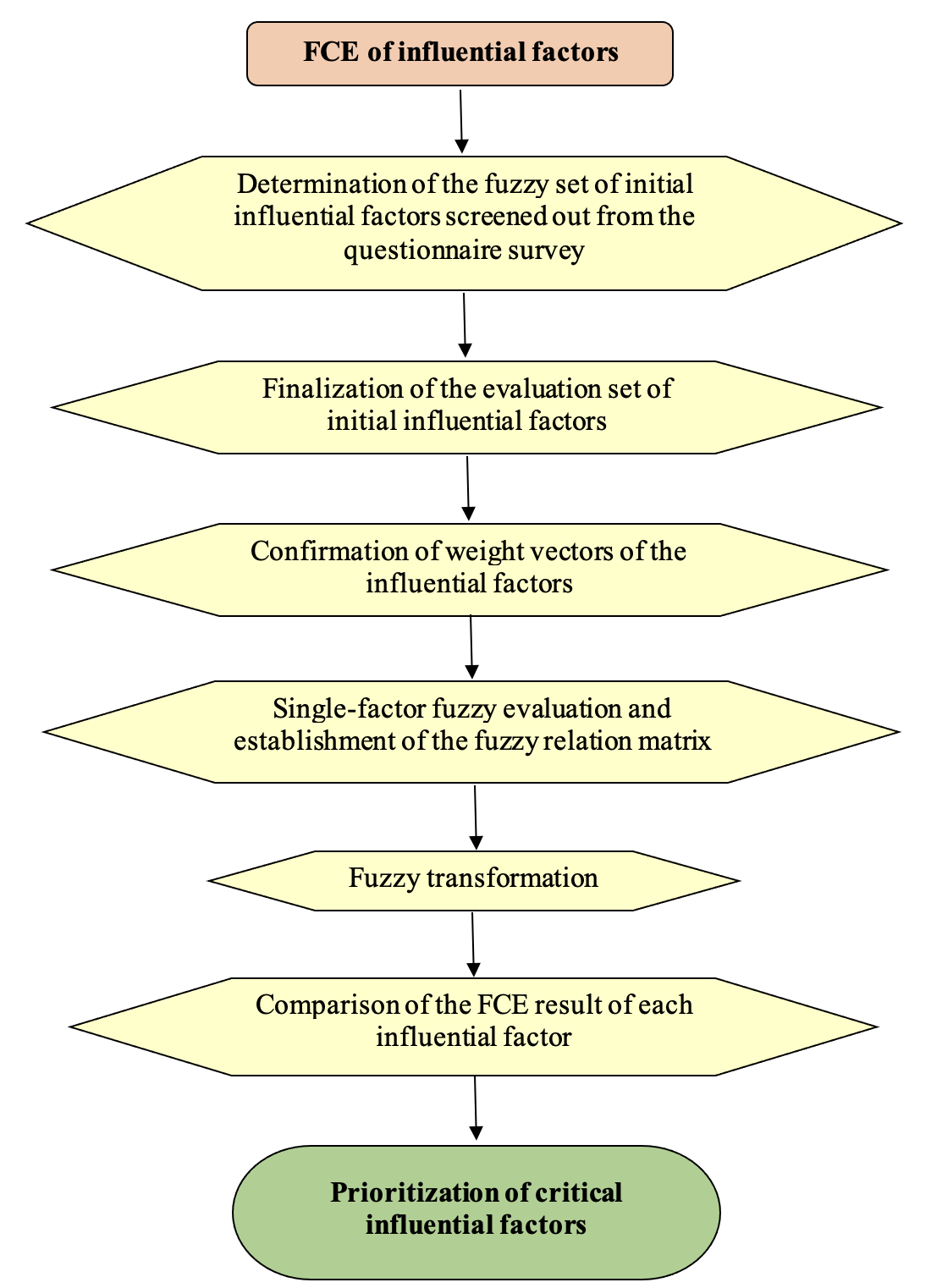 |
Step 1, the fuzzy set of initial influential factors screened out from the questionnaire survey was determined as X = {x1, x2, x3, x4, x5} = {thermal challenge, inside clutter, contaminants emission character, operation process, TPM of blowers and valves}.
Step 2, the evaluation set of initial influential factors was proposed based on economic viability and technological feasibility. Meanwhile, excessive number of elements in the evaluation set should be avoided as this might lead to minimization of the weight coefficient, and consequently poor resolution of membership degrees and difficulty in prioritization of influential factors due to ultra-fuzzy phenomenon [36]. Thus, the evaluation set of initial influential factors was defined as U= {u1, u2, u3, u4, u5} = {experiment complexity, economic cost, environmental pollution, modelling complexity, factor quantifiability}.
Step 3, the weight vectors of each element in the evaluation set were derived from expert panel discussion as A= {a1, a2, a3, a44, a5} = {0.25, 0.2, 0.2, 0.1, 0.25}.
Step 4, the integrated selectivity set could be determined in a relatively straightforward way as V= {v1, v2} = {good, poor}. Based on the single factor fuzzy mathematics evaluation data in Table 2, the following fuzzy matrices of the integrated selectivity for each influential factor were established.
Step 5, the weight averaged composite operator M was selected for fuzzy composition and transformation, and the whole process could be explained conceptually using the following equation (2):
where: bj, the calculated vector of fuzzy comprehensive evaluation; ai, the weight vector of each element in the evaluation set in Step 3; and rij, the element in the fuzzy matrix of the integrated selectivity for each influential factor in Step 4.
The merit of this weight averaged composite operator lies in its combination of both weight coefficient and fuzzy relationship, which could express the interactive effect of multiple influential factors more accurately. The vector of fuzzy comprehensive evaluation for each influential factor was calculated specifically based on the equation (3) below:
where n (1, 2, 3, 4, 5) represents for the five initial influential factors screened out from the questionnaire survey.
Thus, as for the thermal challenge,
likewise, the vectors of fuzzy comprehensive evaluation for inside clutter, contaminants emission character, operation process, and TPM of blowers and valves are listed below, respectively.
it is plausible to normalize this fuzzy vector according to equation 4 below because the sum of elements in the vector is not equal to one (0.525 + 0.555= 1.08).
Through normalization, B3 = (0.486, 0.514);
Lastly, thermal challenge and inside clutter demonstrate optimal fuzzy membership (84.5% and 89%, respectively), while the fuzzy membership of contaminants emission character, operation process, TPM of blowers and valves are relatively low (48.6%, 30% and 39.5%, respectively). Based on the maximum membership principle, thermal challenge and inside clutter are prioritized as the target influential factors for further evaluation of fume hood containment performance. Nevertheless, it is noteworthy that the fuzzy membership of contaminants emission character is much higher than those of operation process and TPM of blowers and valves, which means that contaminants emission character might need to be considered if it interacts with another factor with high membership in real laboratory environments.
Discussion and Conclusion
In this study, previous research of influential factors on fume hood containment performance were reviewed systematically in terms of field test and numerical simulation, which facilitated a better overview of the most common influential factors. A comprehensive survey questionnaire was proposed afterwards in order to collect semi-quantitative data regarding influential factors on fume hood containment performance from the perspective of end-users and experts. With these supportive references, the authors explored the feasibility of selecting influential factors of fume hood containment performance through questionnaire survey and fuzzy comprehensive evaluation. There are numerous influential factors that lead to negative impacts on fume hood containment performance, which in turn add difficulties for indoor environmentalists or industrial hygienists to determine critical factors for further analysis. Although researchers could either select target influential factors based on their experience merely or choose some factors randomly, waste of study time and experimental resources is inevitable as factors are not prioritized properly. Particularly, this issue could be exacerbated when a substantial number of tracer gas testing or CFD modelling are conducted for fume hood containment performance evaluation because the former generates large volume of greenhouse gases (GHG) and the latter requires great computational efforts [37,38].
Thanks to the effective questionnaire survey as well as the comprehensive fuzzy evaluation, two influential factors with strong fuzzy membership were identified eventually for fume hood containment performance evaluation, namely thermal challenge (membership degree 84.5%) and inside clutter (membership degree 89%). While there is no applicable approach in selecting influential factors previously, this paper provides a novel quantitative method to prioritize all the factors and select critical ones among them, which makes further evaluation of fume hood containment performance more cost-effective and time efficient.
There are some recognized challenges in the present study, which can nevertheless be addressed in future work. In the fuzzy comprehensive evaluation, for instance, it is difficult to quantify the weight vectors of each element in the evaluation set accurately, albeit we believe the panel judgement renders valuable data for reference. Relatively small number of questionnaire samples due to time and resource limitations might be another drawback for building up the soundness of the survey. Recommendations for future work include, firstly, expand the scope of the survey and try to acquire more questionnaire samples, which can enhance the overall quality of the survey to some extent and provide more useful data for fuzzy evaluation. Additionally, determination of methodologies for fume hood containment performance evaluation based on the survey questionnaire might be a promising extension of the current study because new technologies are emerging increasingly (e.g., laser-assisted or ultraviolet-fluorescence-based visualization, particle image velocimetry (PIV) measurements [39-45]), and it is not a simple process for selection of optimal methods. Last but not least, it is worthwhile to develop a quantitative algorithm in the future through which the weight vector of each element in the evaluation set can be define more accurately and less subjectively.
Acknowledgments
The authors thank experts from ESIS, Inc., ALS Global, East China University of Science and Technology, University of Saskatchewan, Tongji University, ShanghaiTech University and Shanghai Maritime University who actively participated in the questionnaire survey. Ada Cheng from MESCURE Safety and Technology Institute and Lou DiBerardinis from MIT are highly appreciated for their preliminary review and technical guidance on the design and delivery of the survey instrument.
References
- American Society of Heating, Refrigerating and Air-Conditioning Engineers. Method of Testing Performance of Laboratory Fume Hoods, ASHRAE, Atlanta, GA, 2016. (ANSI/ASHRAE 110).
- Scientific Equipment and Furniture Association. Guide to Selection and Management of Exposure Control Devices in Laboratories (Version 3), SEFA Publications, Garden City, NY, 2017.
- Fuente A, Qiu W, Zhang M, Xie H, Kardous CA, Campo P, Morata TC. Use of the kurtosis statistic in an evaluation of the effects of noise and solvent exposures on the hearing thresholds of workers: An exploratory study. J. Acoust. Soc. Am., 2018, 143(3), 1704. DOI: 10.1121/1.5028368.
- Chen K, Yang JL, Zhang HB, Zhang WJ. Low level noise analysis in laboratory fume hood. J. Chem. Health Saf., 2017, 24(1), 2–7. DOI: 10.1016/j.jchas.2016.03.002.
- Chen K, Zhang HB, Zhang WJ. Measures to alleviate fume hood noise. Am. Lab., 2018, 50(3), 10-13.
- Ahn K, Susan W, Louis DB, Michael E. A Review of Published Quantitative Experimental Studies on Factors Affecting Laboratory Fume Hood Performance. J. Occup. Environ. Hyg., 2008, 5(11), 735-753. DOI: 10.1080/15459620802399989.
- ACGIH. Industrial Ventilation: A Manual of Recommended Practice, ACGIH Publications, Cincinnati, OH, 2004.
- Burgess WA, Ellenbecker MJ, Treitman RD in Ventilation for Control of the Work Environment, 2nd ed., John Wiley & Sons, Inc., Hoboken, NJ, 2004, pp 220–224.
- Koenigsberg J. How does your fume hood rate? New assessment of current fume hood design and operations. J. Chem. Educ., 1992, 69(5), 408-412. DOI: 10.1021/ed069p408.
- Chen K, Wang WZ, Zhang WJ. Investigation of influential factors on laboratory fume hood containment performance. Sci. Technol. Built Environ., 2020, 26(3), 387-399. DOI: 10.1080/23744731.2019.1637192.
- Johnston JD, Chessin SJ, Chesnovar BW, Lillquist DR. The effect of thermal loading on laboratory fume hood performance. Appl. Occup. Environ. Hyg., 2000, 15(11), 863–868. DOI: 10.1080/10473220050175751.
- Chessin SJ, Johnston JD. Thermal loading as a causal factor in exceeding the 0.1 PPM laboratory fume hood control level. Appl. Occup. Environ. Hyg., 2002, 17(7), 512–518. DOI: 10.1080/10473220290035769.
- Ahn K, Ellenbecker MJ, Woskie SR, DiBerardinis LJ. A new quantitative method for testing performance of in-use laboratory chemical fume hoods. J. Chem. Health Saf., 2016, 23(4), 32–37. DOI: 10.1016/j.jchas.2015.10.021.
- He XJ, Steven EG. Quantitative Evaluation of the Performance of an Industrial Benchtop Enclosing Hood. J. Occup. Environ. Hyg., 2013, 10(8), 409-418. DOI: 10.1080/15459624.2013.800466.
- Michele P, Alessio S. A numerical method for the efficient design of free opening hoods in industrial and domestic applications. Energy, 2014, 74: 484–493. DOI: 10.1016/j.energy.2014.07.014.
- Hu PX, Ingham DB, Wen X. Effect of the location the exhaust duct, an exterior obstruction and handle on the air flow inside and around a fume hood. Ann. Occup. Hyg., 1996, 40(2), 127–144. DOI: 10.1016/0003-4878(95)00071-2.
- Fletcher B, Johnson AE. Containment testing of fume cupboards- I methods. Ann. Occup. Hyg., 1992, 36(3), 239-252. DOI: 10.1093/annhyg/36.3.239.
- Fletcher B, Johnson AE. Containment testing of fume cupboards—II. Test room measurements. Ann. Occup. Hyg., 1992., 36(4), 395-405. DOI: 10.1093/annhyg/36.4.395.
- Kim S, Yang H. Estimation of leakage rate of air from a fume hood in a radioisotope laboratory using CFD simulations. Appl. Radiat. Isot., 2018, 140, 300-304. DOI: 10.1016/j.apradiso.2018.07.021.
- Hu PX, Ingham DB, Wen X. Effect of baffles and a louvered bypass on the airflow and the convective patterns of contaminant inside a fume hood. Am. Ind. Hyg. Assoc. J., 1998, 59(5), 303–312. DOI: 10.1080/15428119891010550.
- Sam KY, Lee SH, Ban ZH. Computational fluid dynamics (CFD) modelling on effect of fume extraction. J. Chem. Health Saf., 2019, 26(6), 20-31. DOI: 10.1016/j.jchas.2019.04.004.
- Sławomir P, Piotr K, Michał P. Experimental and numerical flow analysis and design optimization of a fume hood using the CFD method. Chem. Eng. Res. Des., 2018, 132, 627-643. DOI: 10.1016/j.cherd.2018.02.011.
- Kolesnikov A, Ryan R, Walters DB. Use of CFD to design containment systems for work with hazardous materials. Chem. Health Saf., 2003, 10(2), 17–20. DOI: 10.1016/S1074-9098(02)00456-2.
- Flynn MR, Sills ED. On the use of computational fluid dynamics in the prediction and control of exposure to airborne contaminants—An illustration using spray painting. Ann. Occup. Hyg., 2000, 44(3), 191–202. DOI: 10.1016/S0003-4878(99)00091-5.
- Durst F, Pereira JCF. Experimental and numerical investigations of the performance of fume cupboards. Build. Environ., 1991, 26(2), 153-164. DOI: 10.1016/0360-1323(91)90022-4.
- Nichnolson GP, Clark RP, Calcina-Goff ML. Computational fluid dynamics as a method for assessing fume cupboard performance. Ann. Occup. Hyg., 2000, 44(3), 203-217. DOI: 10.1016/S0003-4878(99)00086-1.
- National Oceanic and Atmospheric Administration. Introduction to Survey Design and Delivery, NOAA Publications, Washington, DC, 2015.
- Ogunsola OT, Wang JK, Li S. Survey of particle production rates from process activities in pharmaceutical and biological cleanrooms. Sci. Technol. Built Environ., 2019, 25(6), 692-704. DOI: 10.1080/23744731.2019.1581501.
- Timothy NS, Sang DC, Ahn K. Awareness and perceptions of ergonomic programs between workers and managers surveyed in the construction industry. Work, 2018, 61(1), 41-54. DOI: 10.3233/WOR-182778.
- Zadeh LA. Fuzzy sets. Inf. Control, 1965, 8(3), 338-353. DOI: 10.1016/S0019-9958(65)90241-X.
- Zadeh LA. Fuzzy sets as a basis for a theory of possibility. Fuzzy Set Syst., 1978, 1(1), 3-28. DOI: 10.1016/0165-0114(78)90029-5.
- Zheng GZ, Li K, Bu WT, Wang YJ. Fuzzy comprehensive evaluation of human physiological state in indoor high temperature environments. Build. Environ., 2019, 150, 108-118. DOI: 10.1016/j.buildenv.2018.12.063.
- Mohandes SR, Zhang XQ. Towards the development of a comprehensive hybrid fuzzy-based occupational risk assessment model for construction workers. Saf. Sci., 2019, 115, 294-309. DOI: 10.1016/j.ssci.2019.02.018.
- Muhammad T, Biswajit S. An interactive fuzzy programming approach for a sustainable supplier selection under textile supply chain management. Comput. Ind. Eng., 2021, 155, 107164. DOI: 10.1016/j.cie.2021.107164.
- Hui Z, He XQ, Hani M. Fuzzy comprehensive evaluation of virtual reality mine safety training system. Saf. Sci., 2019, 120, 341-351. DOI: 10.1016/j.ssci.2019.07.009.
- Chen LQ, Zhang P, Ma JL, Wang CL. Fuzzy integrative assessment of oil and gas pipeline risks. Nat. Gas Ind., 2003, 23(2), 117-119.
- California Code of Regulations, Title 8, Section 5154.1: Ventilation Requirements for Laboratory-Type Hood Operations, 2021. Accessed 2021 Jan 31. http://www.dir.ca.gov/title8/5154_1.html.
- American Society of Heating, Refrigerating and Air-Conditioning Engineers, “Determination of Suitable Replacement of SF6 When Used as a Tracer Gas in Accordance with ANSI/ASHRAE Standard 110 (Version 2)”, ASHRAE Report No.: 1573-RP, Atlanta, GA, 2020
- Biraj ST, Dahlhaug OG, Bhola T. Flow measurements around guide vanes of Francis turbine: A PIV approach. Renewable Energy, 2018, 126, 177-188. DOI: 10.1016/j.renene.2018.03.042.
- Xie XM, Claude LM, Nicolas D, Philippe S, Luc F. Local hydrodynamic investigation by PIV and CFD within a Dynamic filtration unit under laminar flow. Sep. Purif. Technol., 2018, 198, 38-51. DOI: 10.1016/j.seppur.2017.04.009.
- Hans HM, Dragana A, Fredrik I, Andreas H. A validation of commonly used CFD methods applied to rotor stator mixers using PIV measurements of fluid velocity and turbulence. Chem. Eng. Sci., 2018, 177, 340-353. DOI: 10.1016/j.ces.2017.11.037.
- Poller B, Hall S, Bailey C, Gregory S, Clark R, Roberts P, Tunbridge A, Poran V, Crook B, Evans C. “VIOLET”: A fluorescence-based simulation exercise for training healthcare workers in the use of personal protective equipment. J. Hosp. Infect., 2018, 99(2), 229-235. DOI: 10.1016/j.jhin.2018.01.021.
- Hall S, Poller B, Bailey C, Gregory S, Clark R, Roberts P, Tunbridge A, Poran V, Evans C, Crook B. Use of ultraviolet-fluorescence-based simulation in evaluation of personal protective equipment worn for first assessment and care of a patient with suspected high-consequence infectious disease. J. Hosp. Infect., 2018, 99(2), 218-228. DOI: 10.1016/j.jhin.2018.01.002.
- Todd B, John S, Justin P, Roger S, Richard J. Ebola virus disease: The use of fluorescents as markers of contamination for personal protective equipment. IDCases, 2015, 2(1), 27-30. DOI: 10.1016/j.idcr.2014.12.003.
- Chen JK, Huang RF, Hsin PY, Hsu CM, Chen CW. Flow and containment characteristics of an air-curtain fume hood operated at high temperatures. Ind. Health, 2012, 50(2), 103-114. DOI: 10.2486/indhealth.MS1326.
|